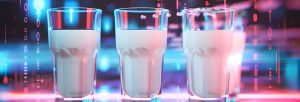
Embracing AI and digital tools to transform dairy R&D is becoming key to accelerating innovation, unlocking insights, and reshaping how new products are processed and developed.
The ongoing digitization of industry processes is transforming operations across a wide range of sectors.
This shift is being driven by the pursuit of increased efficiency, reduced costs, and improved accuracy. Among the most influential developments in this transformation is the rapid advancement of artificial intelligence, which is being integrated into various aspects of business and research.
AI‘s ability to automate complex tasks and generate insights from large datasets makes it particularly valuable in R&D efforts, where it can reduce timeframes and resource demands.
Industries heavily reliant on R&D, such as pharmaceuticals and chemicals, have already begun to implement digital tools to optimize discovery and development of processes. Historically characterized by lengthy and costly R&D phases, these industries are now leveraging AI-driven discovery, modeling, simulation, and data analysis to tackle these challenges.
As the industry continues to explore the potential of digitization, it becomes increasingly important to examine not only the benefits but also the practical and technical considerations involved. Understanding these dimensions is essential for evaluating how digital tools can be harnessed to support breakthroughs in dairy product development and beyond.
Making the most of data
As R&D teams look to improve time-to-market, reduce development costs, and enhance innovation capability, digitization is becoming a critical enabler. Long-established practices are being reshaped by emerging technologies such as cloud infrastructure, predictive modeling, and generative AI.
At Fonterra, one approach that has produced results involves the integration of cloud services with structured data assets. For organizations with extensive research histories, this shift allows decades’ worth of knowledge to be centralized, cleaned, and made more accessible. This makes it possible to build data products: structured, reusable datasets designed to provide insight, support decision-making, and drive modeling efforts.

In parallel, Fonterra is using generative AI to streamline interaction with internal knowledge. Rather than navigating complex documentation, researchers can now pose natural language queries to AI systems grounded on internal data. This can significantly reduce the time required to locate relevant information and improve decision quality across the research lifecycle.
A practical example of this is DairyDetective, a tool built at Fonterra to help scientists instantly search and interpret the more than 17,000 historical research documents created internally. Tools like this – based on an organization’s own research corpus – can summarize findings, and make prior knowledge more actionable in day-to-day innovation workflows.
The power of communication
Digitization delivers several clear benefits across R&D environments. First and foremost is efficiency – digitization allows routine tasks like data retrieval and literature reviews to be streamlined, freeing up more time for experimentation and creative thinking. Knowledge that was once fragmented or difficult to access becomes easier to share and apply, helping to break down silos within teams.
From an R&D-specific perspective, one of the most promising advantages lies in early-stage screening. Learning from the pharmaceutical industry – where generative AI is now used to filter out ineffective compounds before lab trials – dairy researchers can now apply similar techniques to evaluate new formulations or processing methods.
By simulating characteristics like processability or shelf-life performance, digital tools help teams avoid investing in low-potential ideas. This results in better use of research resources and accelerates the pace of development.
Furthermore, digitization can improve cross-functional collaboration. Many R&D projects depend on input from regulatory, commercial, and operations teams. Tools that capture and communicate insights in a clear, contextualized manner help ensure that everyone is aligned, even across geographically distributed teams.
‘Virtual experts’ – AI systems trained and validated by human subject matter experts – can be deployed to answer frequently asked questions and guide users through complex internal processes, making expertize more widely available across an organization, as well as aiding the learning and development process of employees.
Challenges of adoption
While the potential of digital R&D is clear, implementation brings its own set of challenges:
Data Quality The effectiveness of digital tools—particularly those based on AI—depends heavily on the quality of the underlying data. In scientific contexts, inconsistent formats, incomplete records, or outdated terminology can significantly reduce the reliability of AI-generated outputs. Preparing data for use in AI systems often requires considerable effort in data cleaning, validation, and contextual tagging.
Building Trust in AI Systems Another common barrier is trust. Generative AI tools, while powerful, are not immune to ‘hallucinations‘, where they produce plausible-sounding but incorrect information. For research-heavy sectors like dairy, where accuracy is critical, this risk must be managed carefully. Grounding AI models in validated, internally consistent data – and involving subject matter experts in the feedback loop—is key to maintaining confidence in tool outputs.
Adoption Culture Adoption is also influenced by team culture. New tools often face skepticism, especially when they change how people work. The most successful implementations typically involve engaging early adopters – those who are open to trying new approaches and willing to provide constructive feedback. Creating a feedback-rich environment encourages iterative improvement of tools and helps address usability issues early in the rollout.
Embedded into research
The digitization of R&D is not unique to one company or region – it is a shift happening across the global dairy sector and wider industries. The focus is no longer on whether to digitize, but how deeply and how well these technologies are embedded into research operations.
Looking ahead, several trends are shaping the direction of digital R&D. The arrival of next-generation foundational AI models—capable of more nuanced reasoning and contextual understanding—offers new possibilities for literature analysis, hypothesis generation, and design automation.
Alongside this, the concept of data products is also gaining traction. For dairy, this could mean anything from optimizing formulations for specific markets to simulating supply chain constraints in product development. These data-driven approaches allow R&D teams to move faster and make decisions with greater confidence.
Digitization is no longer about future potential – it‘s already reshaping how dairy R&D is conducted. The tools and approaches being piloted today are laying the groundwork for more collaborative, informed, and responsive innovation across the entire industry.
You can now read the most important #news on #eDairyNews #Whatsapp channels!!!
🇺🇸 eDairy News INGLÊS: https://whatsapp.com/channel/0029VaKsjzGDTkJyIN6hcP1K