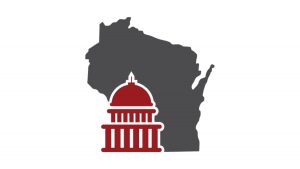
Precision livestock farming is the use of real time monitoring technologies to manage animals by measuring individual data and detecting temporal variability. When it comes to calf care, precision livestock monitoring technologies offer huge potential benefits moving forward. I was at a nutrition conference recently where discussion around the table turned to: can cow people be trained or are those skills that some people are born with?
The ability to look at a slight ear droop or head tilt and know that an animal doesn’t feel well has been critical to farms for years. Combining multiple sensors along with machine learning will allow a virtual cow person to watch every calf all day. These technologies have the potential to detect BRD, which clinically or sub clinically affects almost a third of dairy calves and diarrhea, which clinically affects about 21% of pre-weaned dairy calves.
There are many technologies being developed to assist with calf care and disease diagnosis. Automated feeding systems allow calves to easily be fed more small meals per day compared to most individual manual feeding systems. Producers can set the amount of milk per day a calf will consume or allow ad libitum consumption. These systems more closely mirror nature when calves can drink as much milk as their mothers produce.
These systems collect critical data that can be used for disease diagnosis of drinking speed, number of meals eaten per day, or refusal visits where the calf came to drink but no more milk was allotted for that calf during a set time frame. Both of these factors change when an animal becomes sick due to the natural release of cytokines that cause mammals to feel ill and less interested in eating.
Adding the additional layer of activity data can improve the ability to detect disease in both group and individually housed calves. Some sensor manufacturers are working to identify drinking time of individually-housed calves, but currently tri-axial accelerometers in calves can be used to for primary activity data. This information can be used to determine the number of steps taken a day, the number of lying bouts, the amount of time spent lying, along with the intensity of play activity a calf experiences each day.
All of these factors have been shown to change when a calf becomes sick. The relative changes in these activity measurements are affected by if the calves are group housed or individually housed along with the amount of milk fed causing challenges in alert development. Individually housed calves have an increase in lying time and a decrease in their intensity of activity 3 days prior to disease diagnosis. They also have a significant decrease in step count 2 days prior to diagnosis.
When calves were group housed through these metrics changed. The calves who become ill with diarrhea actually have less lying time and similar activity but more lying bouts. With group housed calves the sick calf often move away from social interactions if lying down when approached by another calf they would get up and move to a different area of the pen.
This interaction increased the number of lying bouts while causing the total amount of lying time and activity to be unchanged. The utilization of machine learning along with a highly trained calf person can allow basic algorithms for disease alerts to learn the how specific farm management affects the way a calf responds when they become ill, creating a dynamic alert for the farm.
The next group of sensors, monitor individual calf temperatures. These sensors can be indwelling in the rumen-reticulum, ear tag mounted to measure ear canal temperature, implanted microchip below the skin, or barn mounted like a thermal imaging camera.
These temperature monitoring technologies are very accurate at measuring the temperature in the location they are in but have been plagued by inaccuracies when compared to the gold standard rectal temperature. They all weakly correlate, increasing when the calf’s rectal temperature increases but not at the same rate or magnitude.
Other technologies don’t have a gold standard to be compared to and the question recently in the precision livestock community is if the correlation of rectal temperature is important or if the ability to relate change to each disease is more important. Utilizing a rumen bolus, we found that the timing of when a calf’s rumen temperature peaks is affected by time of year. These peaks often don’t match the time of day that rectal temperature would normally be taken on the farm, possibly causing a calf with an alert to be marked as healthy.
On weaned calves, temperature monitoring boluses accurately diagnosed 88% of calves who had an alert. These calves were also diagnosed by farm personnel with illness. The rumen bolus fever alert on average was 2.5 days sooner than farm diagnosis. While each of these technologies have shown benefits for disease detection, the future is a system that can layer all of these technologies into an early detection system which learns about the farm, creating a custom alert algorithm.
By Jason Hartschuh, assistant professor, Ohio State University Extension Field Specialist Dairy Management and Precision Livestock.
You can now read the most important #news on #eDairyNews #Whatsapp channels!!!
🇺🇸 eDairy News INGLÊS: https://whatsapp.com/channel/0029VaKsjzGDTkJyIN6hcP1K